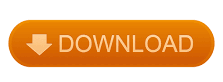
The results of the linear fit are also printed, where x1 is the slope of the power-law tail. On the right is a stack of histograms, showing the distribution of peak intensities for all values of min_delta. On the left is the relationship between the value of min_delta and the number of features in the tree. Variable: y R-squared: 0.962 Model: OLS Adj. run ( verbose = True ) OLS Regression Results = Dep. Importing the dendrograms code, along with a few other common packages: Requires the optional astrodendro package to be installed. The data in this tutorial are available here. The former statistic shows a power-law like turn-off with increasing branch length. 2013 introduced two statistics for comparing the dendrograms of two cubes: the relationship between the number of leaves and branches in the tree versus the minimum branch length, and a histogram comparison of the peak intensity in a branch or leaf. Branches are the connections, while leaves are the tips of the branches.īurkhart et al. The tree structure is comprised of branches and leaves. 2009 to calculate the multiscale properties of molecular gas in nearby clouds. This decomposition was introduced by Rosolowsky et al. In the case of position-position-velocity (PPV) cubes, a dendrogram is a hierarchical decomposition of the emission in the cube. This is known as hierarchical clustering. Plt.In general, dendrograms provide a hierarchical description of datasets, which may be used to identify clusters of similar objects or variables. Plt.title('Hierarchical Clustering Dendrogram (truncated)', fontsize=20) Once with no_plot=True to get the dictionary used to create your label map. The easiest way to set labels is to run dendrogram twice. The leaf_label_func you create must take in a value from R and return the desired label. In addition to creating a plot, the dendrogram function returns a dictionary (they call it R in the docs) containing several lists. You are correct about using the leaf_label_func parameter.
#SCIPY DENDROGRAM HOW TO#
I'm thinking there might be a use here for the parameter 'leaf_label_func' but I'm not sure how to use it. (Note: generating these labels is not the issue here.) I truncate it, and supply a label list to match: labelList = Now let's say I want to truncate to just 5 leaves, and for each leaf, label it like "foo, foo, foo.", ie the words that make up that cluster. To illustrate, here is a short python script which generates a simple labeled dendrogram: import numpy as npįrom import dendrogram, linkage My problem is that, according to the docs, "The labels value is the text to put under the ith leaf node only if it corresponds to an original observation and not a non-singleton cluster." I take this to mean I can't label clusters, only singular points? However, since there can be thousands of words, I want this dendrogram to be truncated to some reasonable valuable, with the label for each leaf being a string of the most significant words in that cluster. I'm using hierarchical clustering to cluster word vectors, and I want the user to be able to display a dendrogram showing the clusters.
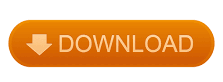